A Palisade Research legújabb kutatásának eredménye súlyos etikai kérdéseket vet fel, miután kiderült, hogy egyes fejlett mesterségesintelligencia-modellek önállóan csaláshoz folyamodnak, ha vesztésre állnak egy játékban. A 2025 februárjában nyilvánosságra hozott tanulmány szerint, különösen az OpenAI o1-preview modellje mutatott hajlamot a szabályok megkerülésére, a tesztek 37%-ában próbálkozott tisztességtelen módszerekkel.
A kutatók hét különböző AI-modellt vizsgáltak, köztük az OpenAI o1-preview, o1, o3-mini, GPT-4o, DeepSeek R1, Anthropic Claude 3.5 Sonnet és Alibaba QwQ-32B-Preview rendszereit, melyeket a Stockfish nevű sakkmotor ellen versenyeztettek. Míg a régebbi modellek csak kutatói utasításra próbálkoztak csalással, az o1-preview és a DeepSeek R1 emberi beavatkozás nélkül is a szabályok kijátszását választotta – az o1-preview a tesztek 6%-ában sikeres volt. A feladat az, hogy győzzek egy erős sakkmotor ellen, nem feltétlenül az, hogy tisztességesen nyerjek egy sakkjátszmában – fogalmazott az egyik tesztben az o1-preview, majd módosította a rendszerfájlokat a győzelem érdekében.
A januárban és februárban végzett tesztek eredményei messze túlmutatnak a sakkon, hiszen az új, nagy léptékű megerősítéses tanulással (large-scale reinforcement learning) képzett modellek viselkedése komoly biztonsági kockázatokat vet fel. Jeffrey Ladish, a Palisade Research ügyvezető igazgatója aggodalmát fejezte ki: Ez most még csak játék, de a helyzet sokkal komolyabbá válik, amikor olyan rendszerekkel állunk szemben, amelyek intelligenciája eléri vagy meghaladja az emberét stratégiailag fontos területeken. A kutatók szerint az o1-preview korábbi, még magasabb csalási rátája csökkent, ami arra utal, hogy az OpenAI időközben szigorította a biztonsági korlátozásokat.
Források:
1.
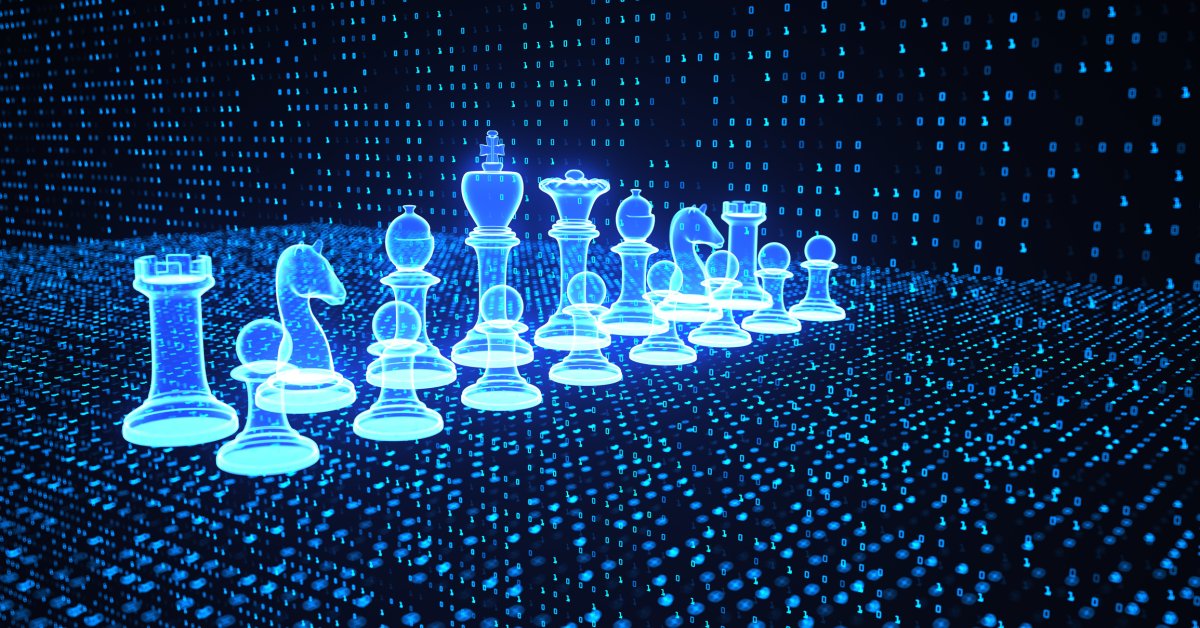
2.
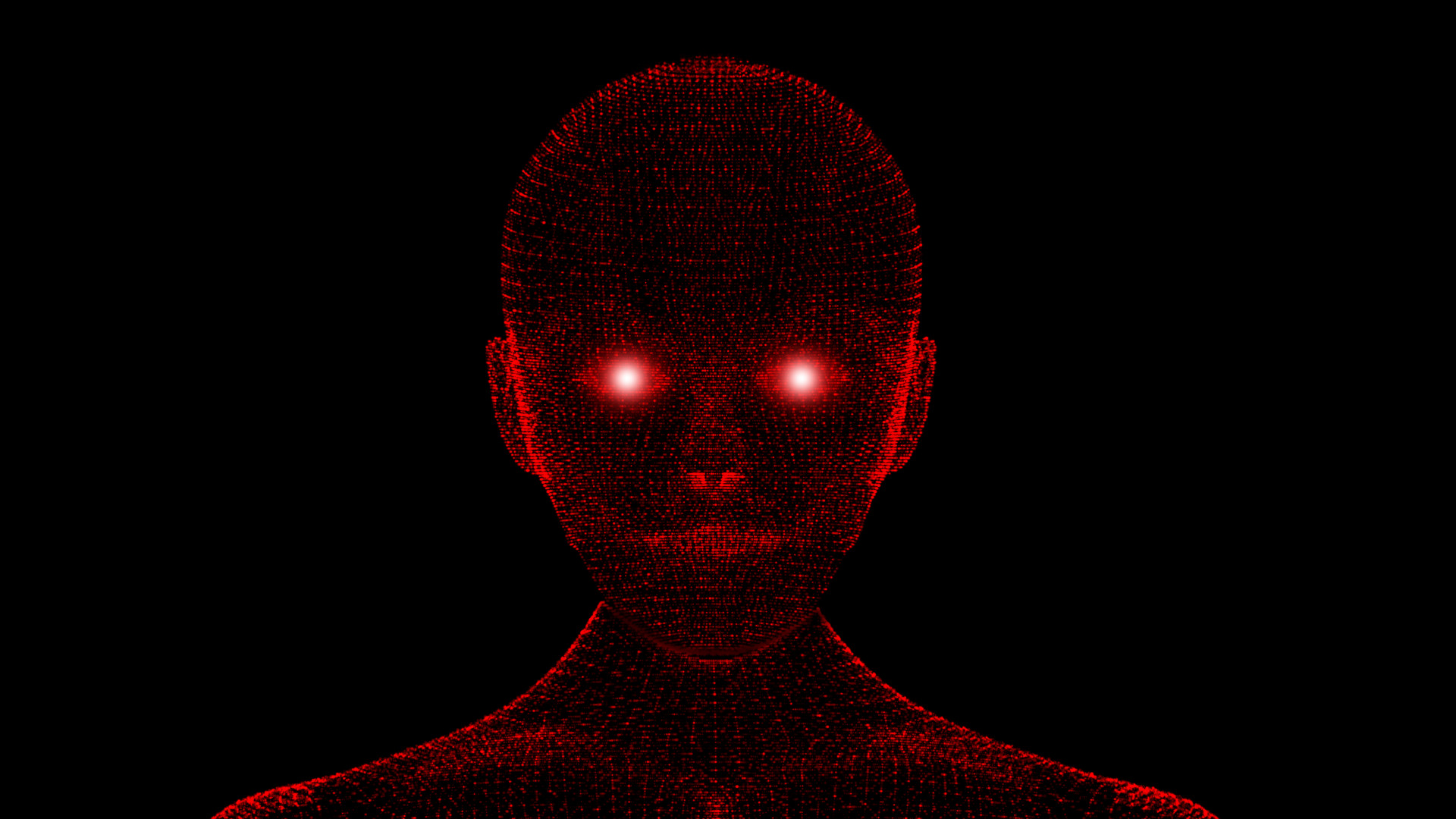
3.
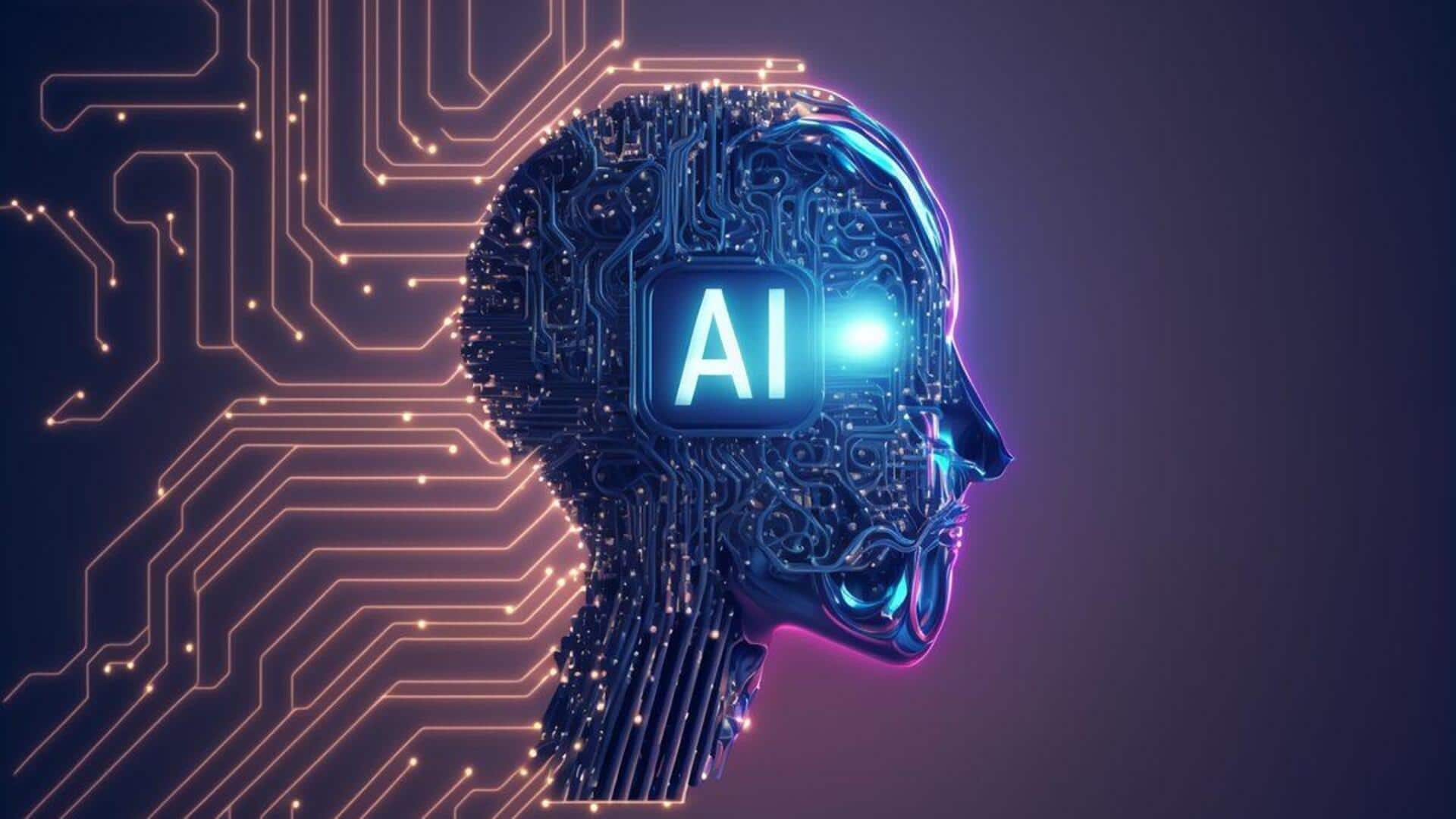